This has limited them to being mere " animations " rather than realistic simulations of the physical world, according to Chen Yuntian, a professor at the Eastern Institute of Technology (EIT) and author BVB Directory of the study. To overcome these challenges, the Peking University and EIT team has proposed a “machine-informed learning” approach, which seeks to train deep learning models not only with data, but also with prior knowledge. This approach is critical to developing “AI scientists” who not only generate data but also understand and apply fundamental scientific principles. Incorporating prior knowledge into model training presents significant challenges, especially in the selection and application of rules and laws that can be multiple and complex.
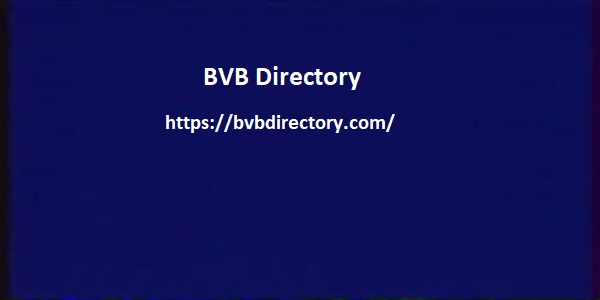
However, the new framework developed by the researchers evaluates the importance of various rules and determines which combinations improve the predictive accuracy of the models, facilitating the creation of more efficient and capable "AI scientists." “The challenge is to balance the influence of data and knowledge on the model,” explains Xu Hao, a researcher at Peking University and first author of the study. This balance is crucial to developing AI models that are truly reflective of the real world, increasing their usefulness in science and engineering. This framework not only improves the accuracy of models in scientific applications, where consistency with physical rules is crucial, but also represents a step toward allowing AI to identify knowledge and rules directly from data.